Timely and reliable population data are crucial for decision-making and planning. Census and household survey data are built on widely used, well-established, rigorous methodologies that have been fine-tuned for many decades. They provide detailed information on populations, and thus are useful for quantifying socio-economic status, among other details, however, the data have limitations:
- Timeliness - Conducting censuses and surveys require a lot of time and resources, so they can’t happen very frequently. Thus, when it is time for analysis, the data are very often too outdated. If new sampling and data collection need to be conducted after disasters or crises, it then takes too long and wouldn’t be available in time to inform emergency response efforts.
- Coverage - Seasonal workers, migrants, and people living in informal settlements are not efficiently captured in census and household survey data. Reaching such highly mobile populations is challenging and they are usually sampled based on residential addresses in the official registration system, which does not account for mobility and current residence. The data are unable to capture the dynamic reality for these groups, such as where they live, if and where they work, whether their children attend or have access to a school, whether they have access to health care, their gender or disability status, ages, and so much more.
- Reliability - A household survey relies on respondent’s ability to recall accurately and this ability generally degrades over time. It affects the reliability of data, particularly when we need longitudinal data over long periods of time.
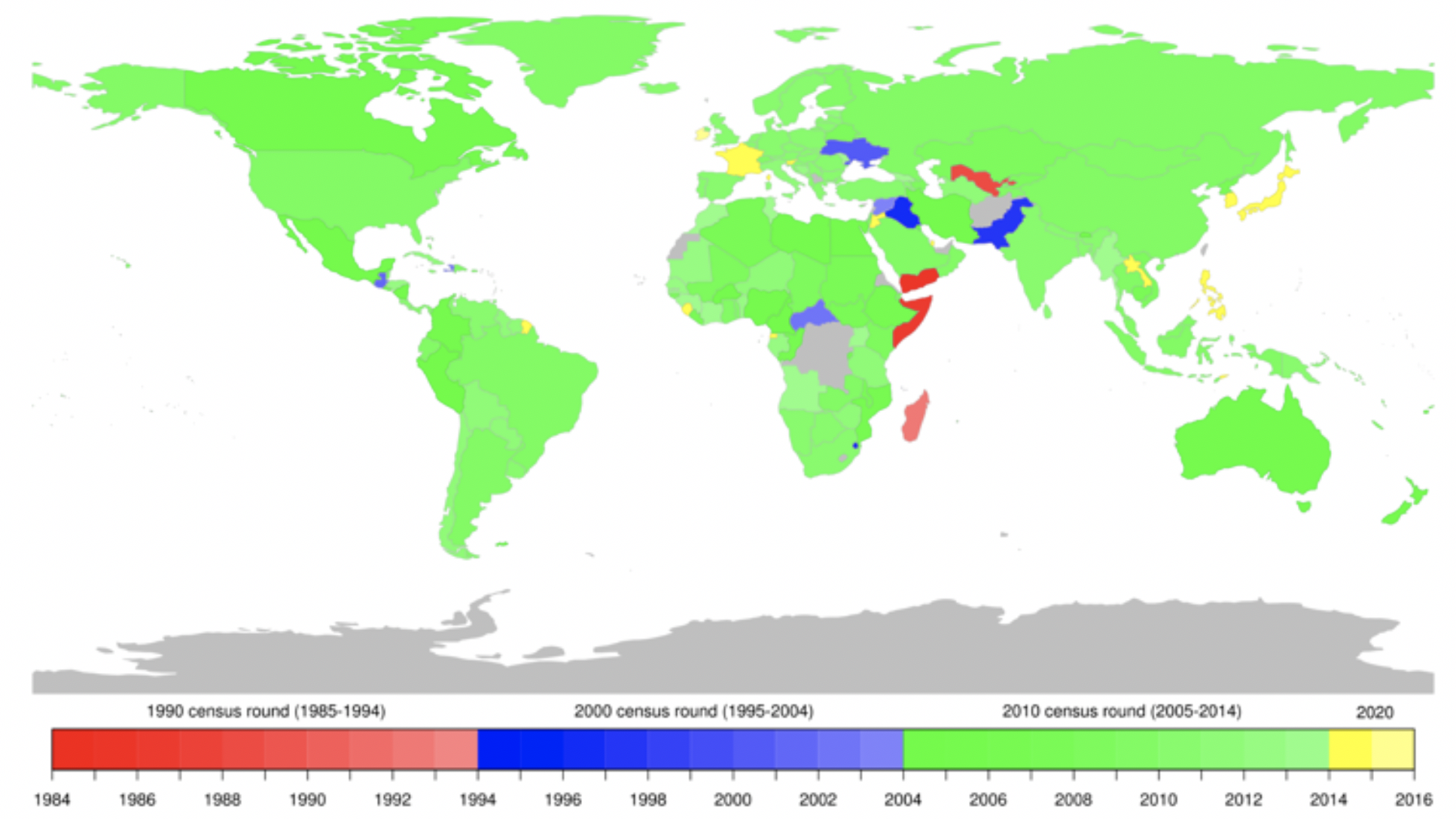
An innovative solution at our fingertips
The University of Tokyo and LIRNEasia, a think tank based in Sri Lanka, developed algorithms to create more frequent and granular population estimates (disaggregated by demography) using telecommunications (telco) data in Sri Lanka. This work required the team to overcome the challenges of using telco data for such purposes. These challenges include non-uniform spatial resolution of the data, inconsistent time intervals between data points, the data’s bias towards people with access to mobile phones, and furthermore, its limitations in what the data can tell us about the population it does represent.
We conducted a smartphone app survey to collect training data for estimating the attributes and adjusting the representativeness bias. We also used data from a large transportation survey, that was conducted by the government, for validation.
The Collaborative Data Innovation Fund, funded by the Trust Fund for Statistical Capacity Building (TFSCB) at the World Bank Group and facilitated by the Development Economics Data Group (DECDG) at the World Bank and the Global Partnership for Sustainable Development Data, provided an opportunity to meet with other project teams to share experiences. Through these meetings, we learned how innovation can impact various layers of society, and discussed different viewpoints around inherent risks and risk mitigation.
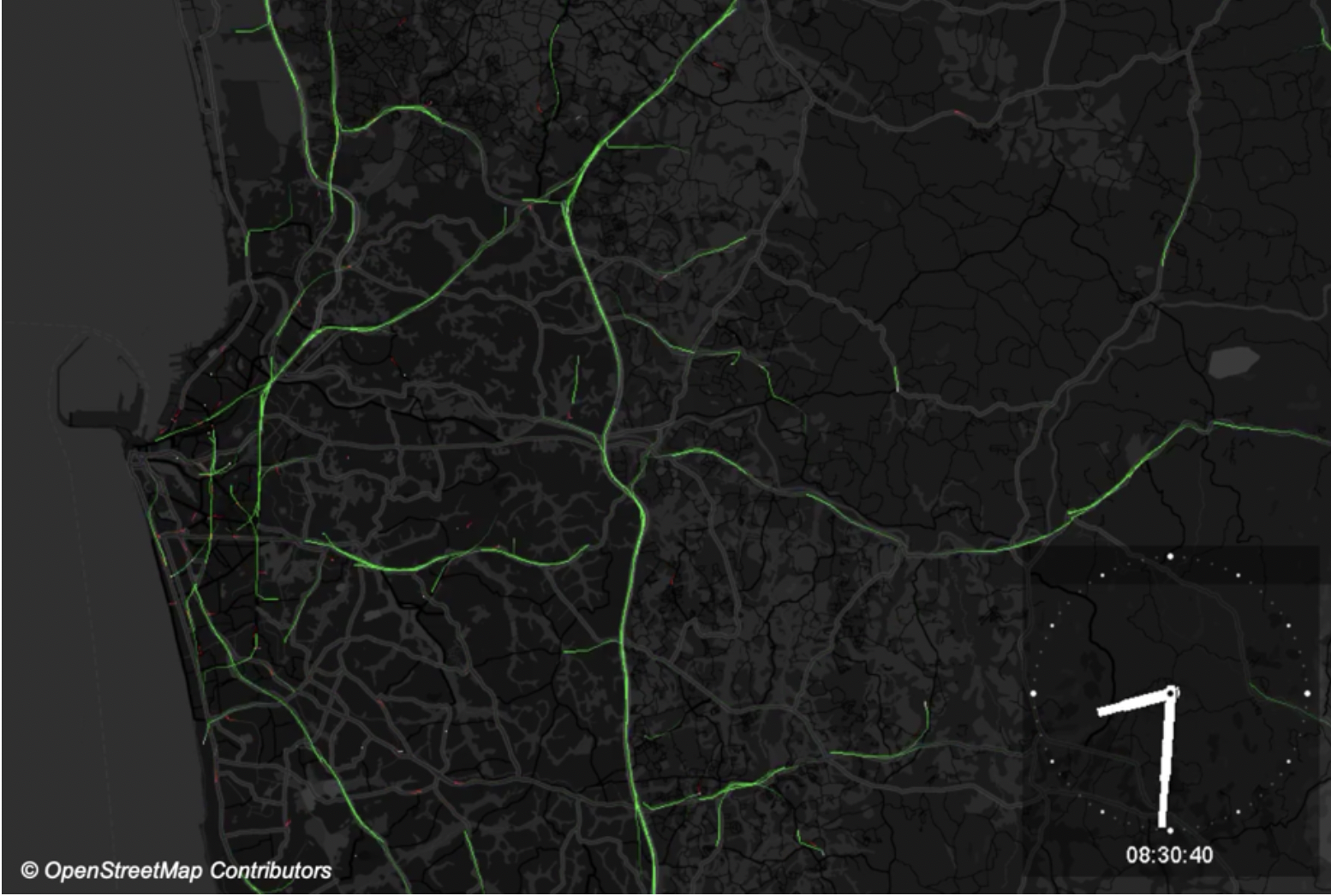
Putting the project to work for COVID-19 response and beyond
Since the algorithms were developed, we have since been able to utilize and implement them in various contexts:
- LIRNEasia was appointed to a government committee for the modernization of the Department of Census and Statistics Sri Lanka (DCS Sri Lanka). In this role, we shared some experiences from this project, including how to complement existing surveys with insights derived from mobile network data, as well as how to deploy specialized apps for data collection.
- Parts of the project’s algorithms were then modified and maintained as open source software within the University of Tokyo’s Spatial Data Commons. Now anyone can access the Mobipack Standalone on GitHub, which can run on ordinary computers and help users analyze and visualize population movements using telco data.
- Using the software, we conducted several trainings for ICT regulators in Mozambique, Rwanda, and in the Gambia as part of an ongoing World Bank project. These trainings were not only for capacity-building, but also for advocating for and showcasing the power of mobile data, thus encouraging local government leadership to utilize new data sources for informing development policy. After one training, for example, we worked with the regulator in Mozambique to examine how telco data can lower the implementation cost of road maintenance surveys. We presented the findings during one of the World Bank’s DECDG Learning Series sessions, “Introduction to Mobile Data: Leveraging Data Access and Technologies for Development Insights,” and held a hands-on training session using the software with fictional telco data.
- We have also subsequently developed Mobipack Hadoop. Mobipack is based on Hadoop - an open source framework that manages data processing and storage for big data applications in scalable clusters - and gives users more flexibility for collecting, processing, analyzing, and managing massive amounts of telco data.
- High-frequency data such as telco data are also proving to be critical for monitoring the rapidly changing population movements caused by COVID-19 mobility restrictions and social distancing. Our system is currently being used by the ICT regulator in two African countries to inform their COVID-19 response efforts. It processes de-identified telco data, maintained securely in the premises of the regulator. The system produces actionable statistics and analysis on population movements that can be used to analyze the spread and impact of the COVID-19.
The population mapping system can be transferred to any other country for use, even now when physical mobility is restricted, as we can support system setup remotely and provide virtual training. We are working remotely with another African country to help them use this system for informing COVID-19 response efforts.
Please visit our website to see how we can support COVID-19 response efforts.
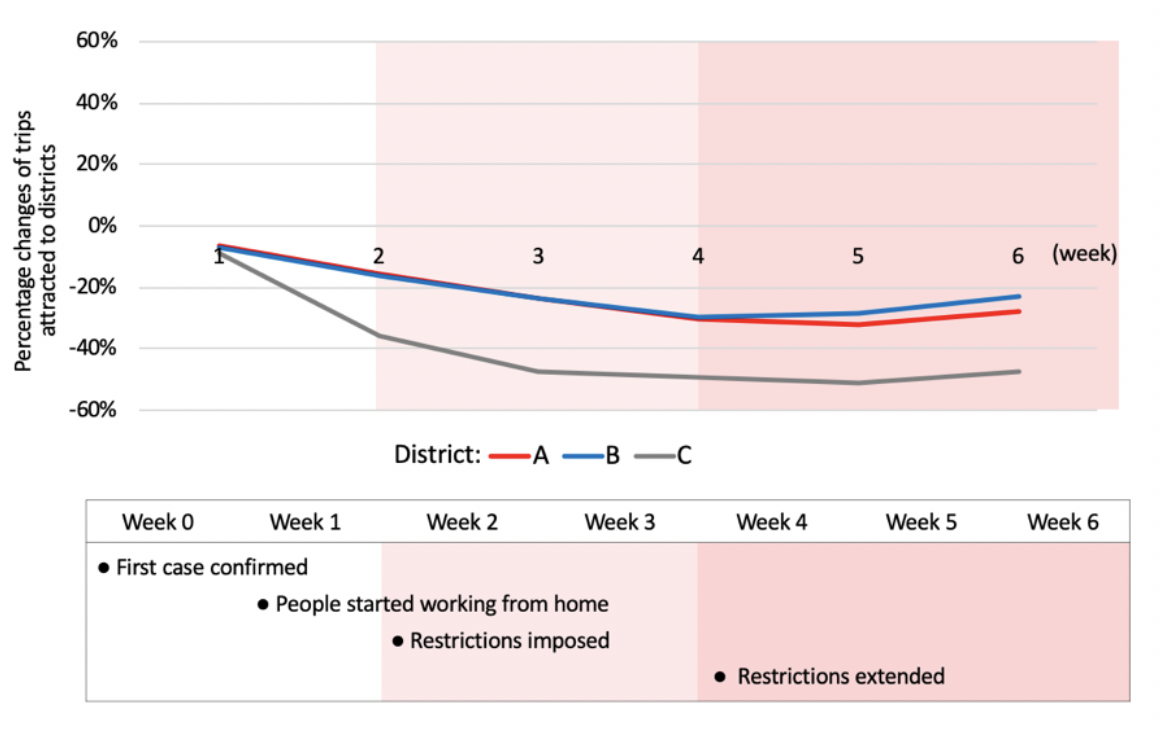
Scaling for further impact
As next steps, we plan to scale this open-source system in other countries and, through capacity-building and trainings, help regulators use it to meet their data needs. The proposed system enables the regulator to process de-identified telco data for extracting useful information as indicators before the data are archived/dumped (data are generally not kept in usable forms for long due to its data size). The system is interoperable and can be used for producing indicators developed by other parties. It enables many low/middle-income countries to produce granular and timely information as indicators using telco data.
Our mission is to empower the global community with new data sources and open technologies. We continue developing open-source tools for processing and analyzing telecom data with use cases. We expect that it can narrow gaps in knowledge, capacity, skills, and incentives for more optimal access and use of new data sources.
The Trust Fund for Statistical Capacity Building III (TFSCB-III) is supported by the United Kingdom’s Department for International Development, the Government of Korea, and the Department of Foreign Affairs and Trade of Ireland.